What is Generative AI and How Does it Work?
Table of Contents
Subscribe To Our Newsletter
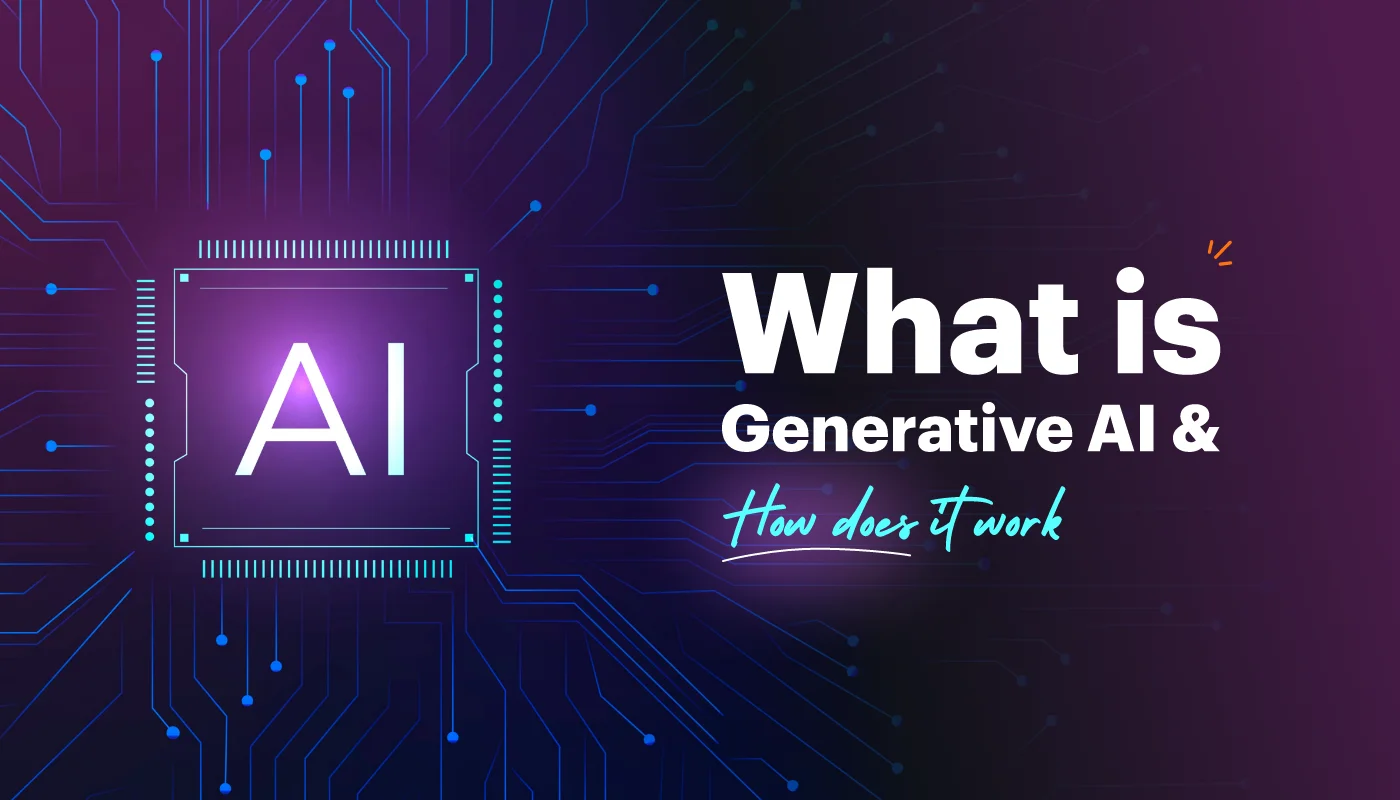
Generative AI stands out as a fascinating and innovative technology. But what exactly it is? How does it weave its magic?
Generative AI is like a smart artist on a computer. It creates new things, like pictures or text, by learning from examples.
- This innovative technology employs intelligent algorithms to produce authentic-looking and sounding content, including images, text, and music.
- Essentially, it’s analogous to having a computer that can exercise creativity and generate content independently.
- The system is designed to function like a classy brain. It draws inspiration from the workings of human-like minds. It utilizes a neural network to analyze and process data.
A fascinating example of Generative AI is the Generative Adversarial Network (GAN), which operates as a pair consisting of a generator and a discriminator in AI technology. The generator produces new things, while the discriminator checks how good they are. They team up and get better at their jobs by challenging each other. It’s like a creative dance between two digital friends.
Types of Generative AI
- Generative Adversarial Networks (GANs)
Imagine a creative duo – the Generator and the Discriminator. GANs bring these two together in a dance of creation. The Generator crafts new things, like images or text, while the Discriminator evaluates how good they are. This constant back-and-forth improves their skills, resulting in realistic and creative outputs. - Variational Autoencoders (VAEs)
VAEs are like artists experimenting with different styles. They learn by trying to reconstruct input data in various ways. This type of Generative AI is great for generating diverse outputs and exploring different possibilities within a given set of data. - AutoRegressive Models
AutoRegressive models are like storytellers predicting what comes next in a sequence. They focus on generating content one step at a time, making them effective for tasks like language generation. GPT (Generative Pre-trained Transformer) models fall into this category, creating coherent text passages by predicting the next word based on context. - Boltzmann Machines
Think of Boltzmann Machines as brainstorming buddies. They consider the relationships between different data points to generate new ideas. This type of Generative AI is often used for collaborative filtering in recommendation systems, suggesting items based on similarities in user preferences. - Transformer Models
Transformers are like multitasking magicians. They can handle different types of data and this quality makes them highly versatile. GPT models, a subset of transformer models, excel in generating human-like text, demonstrating the adaptability of this Generative AI type. - Deep Belief Networks (DBNs)
DBNs are similar to detectives uncovering hidden patterns. They consist of layers that learn to represent complex relationships in data. This type of Generative AI is proficient in tasks like feature learning, making it valuable in uncovering meaningful patterns within large datasets. - Creative Text-to-Image Models
Picture an artist turning words into pictures. Some Generative AI models specialize in transforming text descriptions into images. These models understand textual prompts and generate corresponding visual content, showcasing the intersection of language and image generation. - StyleGAN (Generative Adversarial Networks for Style Transfer)
StyleGAN is like a digital stylist, allowing artists to control the style of generated content. Its ability to transfer artistic styles between images is unparalleled, offering users an unprecedented level of creative influence over the generated outputs. - Recurrent Neural Networks (RNNs)
RNNs are like time-traveling storytellers. They consider previous information when generating new content, making them suitable for tasks involving sequences, such as predicting the next element in a series. - Conditional Generative Models
Conditional Generative Models are like artists taking requests. They create outputs based on specific conditions or inputs. This type of Generative AI is valuable when you want the model to generate content tailored to particular requirements.
What is Generative NLP?
Generative NLP stands for Generative Natural Language Processing. It helps in mastering language with AI.
• Generative NLP is a subset of Generative AI that focuses specifically on language.
• Generative NLP is like a digital wordsmith, understanding and generating human-like text.
• GPT models falling under this category demonstrates the language mastery of Generative NLP.
The Power of Transformer Learning Models in Generative AI
At the heart of Generative AI lies the transformer learning model. Unlike traditional models that process data sequentially, transformers excel in parallel processing, making them highly efficient. This architecture allows the model to understand relationships between words and generate coherent and contextually relevant content.
- Transformers operate on the principle of attention. It enables them to focus on specific parts of the input sequence while generating output.
- This mechanism empowers the model to capture long-range dependencies in data, a key factor in producing high-quality and contextually rich outputs.
- Generative AI, powered by transformer learning models, is transforming how computers generate content.
- These models, like OpenAI’s GPT-3.5, are built on the Transformer architecture, excelling in language tasks and beyond. Transformers stand out for their efficiency and processing information simultaneously.
- They break down data into tokens. It helps in understanding context and relationships within sequences. And, this is very important to grasp the tone of language.
Key Components of Transformers in Generative AI
- Self-Attention Mechanism
Central to Transformers is the self-attention mechanism, a mechanism allowing the model to weigh different words in a sequence differently based on their relevance. This attention to context enables the model to capture dependencies and relationships within the data, facilitating a more nuanced understanding of language. - Multi-Head Attention
Multi-head Attention extends the self-attention concept by employing multiple attention heads, each focusing on different aspects of the input sequence. This parallelized attention mechanism enhances the model’s ability to capture diverse patterns and dependencies, contributing to its overall effectiveness in language-related tasks. - Positional Encoding
While Transformers inherently lack sequential information, positional encoding is introduced to provide the model with an understanding of token positions in a sequence. This addition ensures that the model recognizes the order of words, addressing a limitation of the original Transformer architecture.
The Power of Language Models in Generative AI
Language models, a subset of Generative AI, specialize in understanding and generating human-like text. They are trained on vast datasets, learning the tones of language, grammar, and context. This training prepares them to respond intelligently to prompts, generate coherent text, and even complete sentences.
- A prime example – GPT-3.5, boasts 175 billion parameters. This makes it one of the most potent language models.
- This vast parameter count enables it to understand and generate text across a wide range of topics.
- These models go beyond mere understanding. They produce coherent and contextually relevant text.
- It’s like having a virtual writer who can compose articles, stories, or even poetry.
Autoregressive Models and Autoencoder Models are the two prominent types of language models.
• Autoregressive models generate output one step at a time.
• Autoencoders work by encoding input data into a compact representation and then decoding it to generate output.
Both approaches contribute to the diversity and richness of language generation.
The impact of language models extends to various sectors. In healthcare, these models assist in analyzing medical texts and improving communication. In customer service, they automate responses and so, enhance efficiency. In the education sector, educational tools benefit from language models that give personalized learning experiences.
Despite their benefits, language models raise ethical concerns. They may unintentionally spread biases present in the training data. Thus, ensuring fairness and accountability in their use becomes crucial.
Key Components of Language Models in Generative AI
- Attention Mechanism
At the core of language models is the attention mechanism. This mechanism allows the model to weigh different words in a sequence differently based on their relevance. By attending to specific parts of the input sequence, the model can capture dependencies and contextual nuances. It facilitates a more sophisticated understanding of language. - Contextual Embedding
Contextual embeddings are used by language models to represent words by considering the context in which they appear. In contrast to traditional word embeddings that assign a static vector to each word, contextual embeddings adjust their representation based on the surrounding words in a specific context. This dynamic approach enhances the model’s capacity. After which, they capture the changing meaning of words in various contexts. - Recurrent Neural Networks (RNNs)
Language models are typically developed using RNN or Transformers. RNN operates by processing sequences incrementally and retaining a hidden state that preserves information from previous steps. On the contrary, Transformers excel in parallel processing. It enables more efficient handling of sequential data. The selection of these architectures is reliant upon the certain demands of the given task.
Let’s decode the difference between transformer learning models and language models below.
Transformer Learning Models VS Language Models
- While transformer learning models are the backbone of Generative AI, language models serve as its expressive voice.
- With the parallel processing efficiency, Transformers lay the groundwork for quick learning. While language models are exemplified by GPT-3.5. They can take this efficiency to creative heights.
- The key difference lies in their focus: transformers focus on data processing efficiency and context understanding, while language models focuses on language generation.
- Transformers, with their attention mechanism, excel in capturing complex relationships within data. This makes them versatile for various AI applications beyond language generation. On the other hand, Language models specialize in understanding and generating human-like text. This makes them adept storytellers and conversationalists.
Final Takeaway
The possibilities of Generative AI seem endless. From reshaping design to revolutionizing how we communicate through language; Generative AI is such worthwhile technology for uncharted creative territories.
However, like any powerful tool, Generative AI comes with responsibilities. Addressing issues like bias and privacy ensures that the magic of Generative AI contributes positively to our digital world. Thus, it fosters a creative revolution that is beneficial for all.
Unleash the power of tomorrow with Generative AI technology – where innovation meets possibility. Join hands with leading Generative AI companies like Codiant to shape a future powered by limitless creativity.
Frequently Asked Questions
Generative AI is an artificial intelligence model that can create new data. For example, it can create text, images, sound, or even computer code. It gets its knowledge from the data it’s trained on. This AI uses this information to make fresh, original content that has never existed before.
Suppose you give this type of AI an input, like a piece of text or a picture. It then uses deep learning to make an output similar to or a continuation of the input. It may use neural networks, transformer models, or diffusion models to do this. For instance, think about language models like ChatGPT, or image generators like DALL-E or Stable Diffusion
ChatGPT is a language model that has been trained on huge amounts of text from the internet. Also many examples are Google Bard, Bing AI, and Jasper etc
ChatGPT, specifically, is a large language model trained on a vast amount of text data from the internet. It uses transformer architecture and self-attention mechanisms to understand the context and relationships within the input text, allowing it to generate coherent and relevant responses while maintaining a conversational flow.
Featured Blogs
Read our thoughts and insights on the latest tech and business trends
Top Benefits Of The Metaverse In Healthcare
- May 14, 2024
- Technology
The Metaverse is changing healthcare by offering immersive experiences that improve medical training, patient engagement, and research. It connects healthcare professionals with patients remotely and provides interactive virtual clinics for personalized care. Medical providers can... Read more
Machine Learning In Healthcare: Applications, Benefits & Future Trends
- April 19, 2024
- Technology
The way we take care of people's health is changing swiftly! Instead of just using tools like scalpels and stethoscopes, doctors are now using super smart computer programs called artificial intelligence, especially one called machine... Read more
Overcome Digital Transformation Challenges in Large Organizations
- April 11, 2024
- Technology
Change is happening fast in the digital world, and for large organizations, it's like climbing Mount Everest – tough, challenging, but definitely worthwhile. Don't worry, though! Even though the journey to digital transformation can be... Read more