Machine Learning In Healthcare: Applications, Benefits & Future Trends
Table of Contents
Subscribe To Our Newsletter
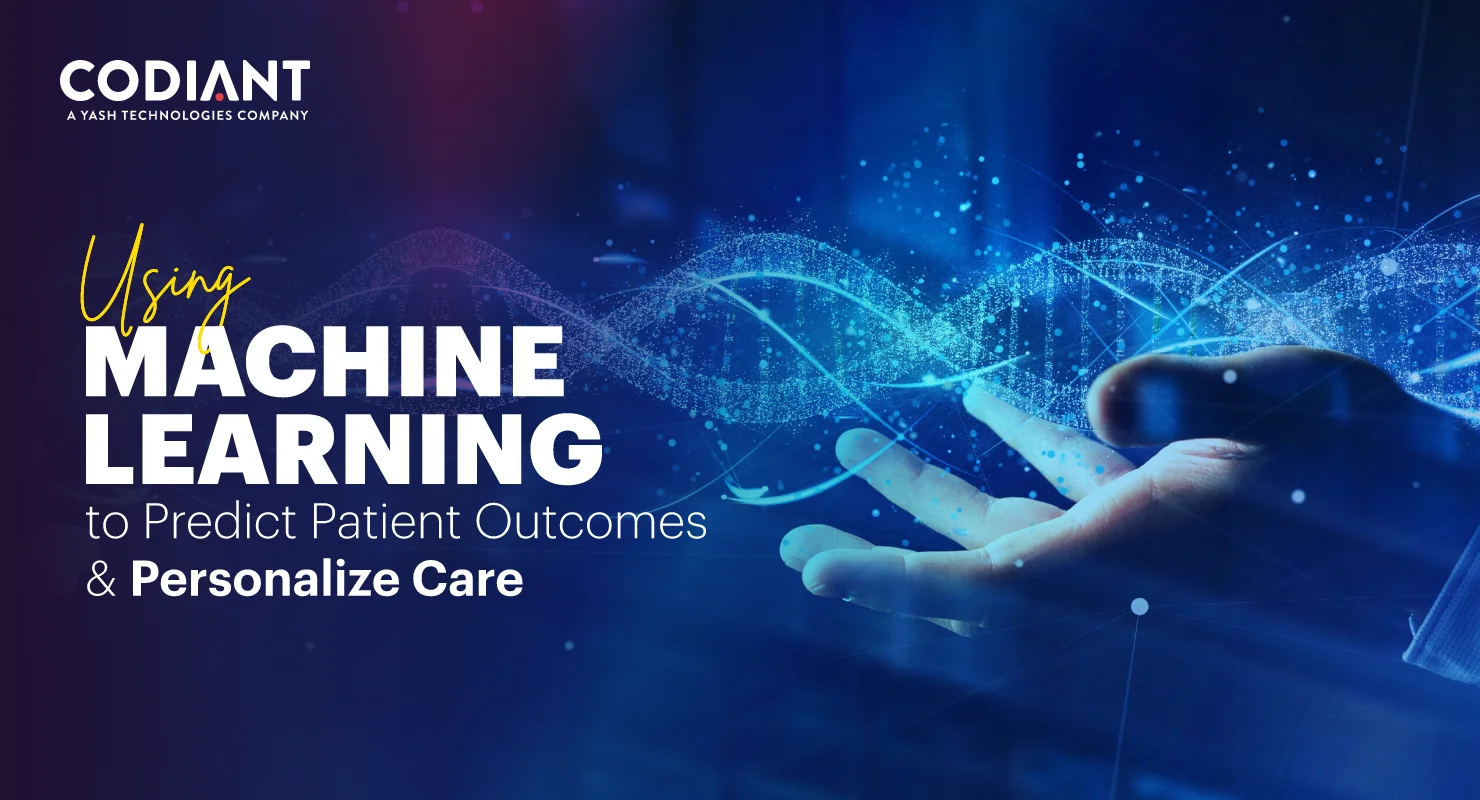
The way we take care of people’s health is changing swiftly! Instead of just using tools like scalpels and stethoscopes, doctors are now using super smart computer programs called artificial intelligence, especially one called machine learning.
These programs are helping in lots of different ways to make patients healthier. In this blog, we’re going to talk about how beneficial artificial intelligence is & how machine learning in healthcare revolutionizing the industry.
We’ll also explore its applications, the multitude of benefits it offers, and a glimpse into the future trends that will shape this transformative technology.
A Glimpse into the Future of Medicine
Machine learning isn’t just a far-off dream; it’s already changing healthcare as we speak. Whether it’s helping doctors diagnose diseases faster or making paperwork easier, machine learning is giving healthcare workers the tools to provide better, more tailored care.
However, we’re only scratching the surface here. As we explore further, you’ll see how machine learning algorithms are set to transform everything from finding new drugs to keeping an eye on patients, leading to a healthier future for everyone.
Why Machine Learning Matters In Healthcare
The human body is really complicated, and regular healthcare methods sometimes struggle to keep up. But that’s where machine learning comes in! It’s like having an incredibly sharp medical assistant that looks at tons of medical information and finds things that humans might miss.
This helps doctors find diseases earlier and make personalized treatment plans for each person based on their own medical history. Plus, it saves time by doing boring paperwork, so doctors can spend more time helping patients.
Machine learning is becoming a big deal in modern medicine because it’s making healthcare better and more efficient. Let’s explore together how this amazing technology is changing healthcare and what we might see in the future!
Machine Learning: A Primer
Before we explore healthcare apps more, let’s first understand the basics of machine learning in simple terms.
What is machine learning?
Machine learning is a part of AI that works on making algorithms capable of learning from data without being programmed directly. Unlike regular software that needs step-by-step instructions, machine learning gets better by learning from experience. It learns from big sets of data, figuring out patterns and connections within it. This knowledge helps it make predictions or decisions when faced with new data it hasn’t seen before.
Understanding the Mechanics of Machine Learning
Machine learning is a process where computers learn from data to make decisions or predictions. First, a lot of relevant data, like patient records and medical images, is gathered. This data needs to be cleaned up and organized.
Then, a specific computer method is chosen and taught using this data. It keeps looking at the data, adjusting itself to get better at spotting patterns. After that, its performance is checked on different data to see how well it works.
If needed, it gets fine-tuned. Once it’s good enough, it’s used to make predictions on new data. There are different types of methods, like supervised learning, where the computer learns from labelled examples, and unsupervised learning, where it finds patterns in data without labels.
These methods are changing healthcare by helping us diagnose diseases better and personalize treatments.
Data and Facts to Consider
- A study by Accenture found that AI has the potential to generate $150 billion annually in savings for the US healthcare system by 2026.
- The global market for AI in healthcare is expected to reach $67.6 billion by 2025 [source needed].
- A survey by PWC found that 80% of healthcare executives believe AI will significantly transform their organizations in the next five years by statista.
- A fifth of healthcare organizations have already adopted some form of AI.
- AI could reduce the cost of discovering new drugs by 70%.
- Predictive AI tools could reduce hospital admissions by half.
Applications Of Machine Learning In Healthcare
Machine learning is transforming healthcare by offering a powerful set of tools for diagnosis, treatment, and beyond. Let’s explore some key applications:
Patient Diagnosis
Machine learning algorithms can analyse vast amounts of medical data, including patient records, imaging scans, and lab results, to identify patterns and anomalies that might indicate disease. This can significantly aid in:
- Early Detection: Machine learning algorithms have the ability to detect subtle changes in data that might go unnoticed by human doctors. This can result in earlier diagnoses and better treatment outcomes. For example, there are algorithms being created to analyse mammograms for indications of breast cancer. This could lead to detecting cancer earlier, potentially increasing survival rates for patients.
- Differential Diagnosis: When a patient has multiple symptoms, machine learning can analyse various possibilities and recommend the most probable diagnoses, simplifying the diagnostic process.
Real-World Example: IBM’s Watson Oncology is a machine learning platform that examines a patient’s medical history, genetic information, and tumour traits to propose personalized treatment options. It does this by drawing insights from successful past cases.
Treatment Personalization
One size doesn’t fit all in medicine. Machine learning allows for the creation of personalized treatment plans by:
- Analysing Patient Data: Machine learning algorithms can consider a patient’s individual medical background, genetic composition, and lifestyle elements to forecast how they may react to various treatments.
- Optimizing Treatment Regimens: Using this analysis, machine learning can recommend personalized treatment strategies that are more likely to be successful for each individual patient. This personalized approach may help minimize side effects and enhance treatment outcomes.
Real-World Example: Initially, Iressa, a drug for lung cancer, didn’t work well for many patients. However, researchers utilized machine learning to analyse genetic information. This allowed them to pinpoint patients with particular mutations who were most likely to respond positively to the drug. As a result, treatments became more targeted, offering better outcomes for patients.
Drug Discovery
The traditional drug discovery process is slow and expensive. Machine learning is accelerating this process by:
- Identifying Drug Targets: Machine learning can go through extensive datasets of biological information to pinpoint potential targets for developing new drugs.
- Simulating Drug Interactions: Machine learning can help predict how well new drugs might work and what side effects they could have by simulating how they interact with the body.
Real-World Example: In the fight against Ebola, researchers used machine learning to analyse the virus’s genetic makeup and identify potential targets for new drugs. This significantly accelerated the development process.
Predictive Analytics
Machine learning can analyse medical data to predict future health events, enabling:
- Disease Outbreaks: By examining past data on elements such as weather conditions and travel habits, machine learning algorithms can forecast the spread of infectious diseases. This enables earlier intervention and implementation of containment strategies.
- Patient Risk Stratification: ML can identify patients at high risk for certain diseases, allowing for preventative measures and early intervention.
Real-World Example: The Mayo Clinic (a healthcare organization based in Rochester, Minnesota, USA) uses machine learning to analyse patient data and predict their risk of developing hospital-acquired infections. This allows for early intervention and improved patient outcomes.
These are just a few examples of how machine learning is revolutionizing healthcare. As this technology continues to evolve, we can expect even more transformative applications that will improve patient care, reduce costs, and ultimately lead to a healthier future for all.
Advantages of Applying Machine Learning in Healthcare
1. Enhanced Diagnostic Accuracy And Early Detection
Machine learning is like a super-smart computer program that learns from loads of medical images to spot diseases really accurately. For instance, it can detect cancer in body scans such as mammograms and X-rays. This helps doctors catch diseases like breast cancer and lung cancer early, which means better treatment options.
In skin care, machine learning is excellent at spotting skin issues, even serious ones like melanomas. This early detection can help doctors treat these problems sooner and could even save lives.
Machine learning can also analyse brain scans to help diagnose brain diseases like Alzheimer’s and Parkinson’s. This early diagnosis means treatment can start sooner, and patients’ conditions can be managed better.
These are just some ways machine learning helps in healthcare. As these smart algorithms keep learning and improving, they’re expected to become even better at diagnosing diseases early, promising a future where diseases can be spotted and treated much sooner.
2. Personalized Medicine: A Tailored Approach To Treatment
Personalized medicine is a modern approach to healthcare that uses machine learning, a type of intelligent computer program. Instead of giving the same treatment to everyone, it considers factors like a person’s medical history and genes to predict how they’ll respond to different medicines and therapies. This helps doctors create treatment plans tailored to each individual, resulting in better outcomes and fewer side effects.
Machine learning also helps determine the right dosage of medicine for each person, optimizing treatment effectiveness and reducing the risk of adverse reactions. Additionally, it can analyse vast amounts of patient data to identify individuals at high risk, enabling doctors to adjust their treatment plans to ensure patient safety.
In essence, combining personalized medicine with machine learning allows healthcare providers to move away from a one-size-fits-all approach, providing truly personalized care that revolutionizes patient treatment and results.
3. Streamlined Workflows And Improved Efficiency
Healthcare workers often have too much paperwork and tasks to handle. Machine Learning (ML) can help by doing some of these tasks automatically. For example, it can schedule appointments, process insurance claims, and make reports without people having to do it manually.
This frees up time for doctors and nurses to take care of patients. ML also helps organize patient information better, so doctors can make better decisions. It can also catch mistakes before they happen, like giving the wrong medicine. Overall, using ML in healthcare makes things easier for workers and helps patients get better care.
4. Accelerated Drug Discovery And Development
Developing new drugs is usually slow and expensive, but now with the help of machine learning (ML), things are changing.
ML can quickly find potential targets for new drugs by looking at lots of biological data. It can also predict how well a new drug might work and if it could cause any problems in the body. ML can even find new uses for drugs that already exist.
This means we might find treatments for diseases faster and at a lower cost. Overall, using ML for drug discovery is exciting and could help us solve health problems faster.
5. Proactive Healthcare And Disease Prevention
Moving beyond just treating illnesses when they happen, machine learning (ML) is helping healthcare become more proactive. ML can predict when and where diseases might spread by looking at past data like weather and travel patterns.
This helps authorities act earlier to stop outbreaks from getting worse. ML also helps identify people who might be at risk of getting sick based on their health records. This allows doctors to suggest things like early check-ups or lifestyle changes to prevent illnesses.
Additionally, ML can give personalized advice on how to stay healthy by looking at your individual health history. This means you get recommendations that are just right for you. Overall, ML is changing healthcare by helping us be more prepared, personalized, and proactive in staying healthy.
Future Trends In Machine Learning For Healthcare
Machine learning (ML) is already revolutionizing healthcare, and its influence is set to expand further in the years ahead. Here’s a glimpse into some exciting upcoming trends and technologies:
1. Enhanced Diagnostics With Ai
- Advanced Image Analysis: In the future, machine learning algorithms will get better at looking at medical images like X-rays, MRIs, and CT scans. They’ll be able to spot diseases earlier and more precisely. Plus, they’ll be able to do tasks like outlining lesions and assessing risks, which will save time for radiologists, letting them focus on more challenging cases.
- Multimodal Learning: Combining data from different places, such as imaging tests, genetics, and electronic health records (EHRs), will give doctors a better understanding of a patient’s health. This will help them make more accurate diagnoses and create treatment plans tailored to each person’s needs.
2. Precision Medicine And Proactive Care
- Predictive Analytics: Machine learning will be pivotal in predicting individual disease risks. By analysing large amounts of data, algorithms can pinpoint patients who are more likely to develop certain conditions, enabling proactive interventions and early treatment.
- Personalized Medicine: Machine learning will customize treatment plans according to a person’s genes, health history, and lifestyle. This personalized approach can make treatments work better and reduce unwanted side effects.
3. Remote Patient Monitoring And Virtual Assistants
- Wearable Devices and Sensors: Machine learning algorithms can keep an eye on data from wearable devices and sensors all the time. They can spot early signs of problems or when a chronic condition is getting worse, which helps in acting quickly.
- AI-powered Chatbots and Virtual Assistants: AI companions will offer patients constant support, reminding them to take medication and answering simple health queries, which can reduce the workload on healthcare providers.
4. Drug Discovery And Development
- Drug Target Identification: Machine learning can go through huge amounts of molecular data to find potential targets for new drugs. This speeds up the process of discovering new medications a lot.
- Clinical Trial Optimization: Machine learning can improve how clinical trials are set up by finding the right people to participate and predicting how the trials will turn out. This means new treatments can be developed more quickly and work better.
5. Public Health And Population Health Management
- Predicting and Monitoring Outbreaks: Machine learning can look at social media posts, travel patterns, and healthcare records to predict and track disease outbreaks right as they happen. This helps us act fast and make sure we have enough resources where they’re needed most.
- Resource Allocation and Policymaking: Insights generated by machine learning can guide public health policies by pinpointing regions where diseases are widespread or resources are lacking. This data-driven method helps distribute healthcare resources more effectively.
Challenges And Considerations
While the future of ML in healthcare is promising, there are challenges to address:
- Data Privacy and Security: It’s crucial to prioritize patient data privacy and security. Strong frameworks for governing data are necessary to ensure this.
- AI Bias and Explainability: Machine learning models can learn biases from the data they use. It’s important to make sure that AI decisions are fair and easy to understand so that people trust and use them.
- Clinical Integration and Regulatory Frameworks: Making sure that machine learning tools fit smoothly into how doctors work and creating clear rules for using them are really important for making them widely used in healthcare.
To Sum Up
Machine learning can totally change healthcare with impact of telemedicine. It can help doctors find problems early, give treatments customized to each person, and make healthcare systems work better. But as we use it more, we need to make sure people’s private health info stays safe, and that the technology is fair to everyone. Also, instead of replacing doctors, it will work with them, helping them make better decisions. It’s not a magic fix, but it can make healthcare more personal and effective for everyone.
Frequently Asked Questions
Yes, machine learning can be safe for patient information. It uses data encryption and privacy regulations to protect patient information. But it’s important to have strong security measures in place.
No, machine learning won’t replace doctors. It’s a tool that can help doctors by providing insights and analysis, but it can’t replace the human touch and expertise of a doctor.
If a machine learning model makes a mistake, it’s usually caught during a review by healthcare professionals. The model can then be adjusted and improved. It’s important to remember that these models are tools to assist, not replace, healthcare professionals.
Yes, patients can see the impact of machine learning on their care through improved diagnosis, personalized treatment plans, and better health outcomes. However, the technical details of how machine learning works might not be visible to them.
The upfront costs can vary. They include costs for data collection and storage, developing and training the machine learning models, and integrating them into existing systems. There may also be ongoing costs for maintenance and updates.
Featured Blogs
Read our thoughts and insights on the latest tech and business trends
Overcome Digital Transformation Challenges in Large Organizations
- April 11, 2024
- Technology
Change is happening fast in the digital world, and for large organizations, it's like climbing Mount Everest – tough, challenging, but definitely worthwhile. Don't worry, though! Even though the journey to digital transformation can be... Read more
Top AI Trends Lighting Up Innovation 2024
- April 10, 2024
- Artificial Intelligence
2024 is set to be a significant year for top AI trends, especially generative AI, following its explosive emergence in 2022 and initial business exploration in 2023. This year, the focus is on making AI... Read more
Why Investing in Mobile App Maintenance Is Important ?
- April 9, 2024
- Mobile App Development
Our daily activities are greatly influenced by using mobile apps! Whether for ordering food, buying apparel, listening to music, playing games, reading news, or transferring money, there is an app available for every need. The... Read more